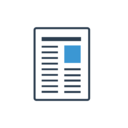
survInTime - Exploring surveillance methods and data analysis on Brazilian respiratory syndrome dataset and community mobility changes


Background The covid-19 pandemic brought negative impacts in almost every country in the world. These impacts were observed mainly in the public health sphere, with a rapid raise and spread of the disease and failed attempts to restrain it while there was no treatment. However, in developing countries, the impacts were severe in other aspects such as the intensification of social inequality, poverty and food insecurity. Specifically in Brazil, the miscommunication among the government layers conducted the control measures to a complete chaos in a country of continental dimensions. Brazil made an effort to register granular informative data about the case reports and their outcomes, while this data is available and can be consumed freely, there are issues concerning the integrity and inconsistencies between the real number of cases and the number of notifications in this dataset.
Results We projected and implemented four types of analysis to explore the Brazilian public dataset of Severe Acute Respiratory Syndrome (srag dataset) notifications and the google dataset of community mobility change (mobility dataset). These analysis provides some diagnosis of data integration issues and strategies to integrate data and experimentation of surveillance analysis. The first type of analysis aims at describing and exploring the data contained in both datasets, starting by assessing the data quality concerning missing data, then summarizing the patterns found in this datasets. The Second type concerns an statistical experiment to estimate the cases from mobility patterns organized in periods of time. We also developed, as the third analysis type, an algorithm to help the understanding of the disease waves by detecting them and compare the time periods across the cities. Lastly, we build time series datasets considering deaths, overall cases and residential mobility change in regular time periods and used as features to group cities with similar behavior.
Conclusion The exploratory data analysis showed the under representation of covid-19 cases in many small cities in Brazil that were absent in the srag dataset or with a number of cases very low than real projections. We also assessed the availability of data for the Brazilian cities in the mobility dataset in each state, finding out that not all the states were represented and the best coverage occurred in Rio de Janeiro state. We compared the capacity of place categories mobility change combination on estimating the number of cases measuring the errors and identifying the best components in mobility that could affect the cases. In order to target specific strategies for groups of cities, we compared strategies to cluster cities that obtained similar outcomes behavior along the time, highlighting the divergence on handling the disease.
SEEK ID: https://workflowhub.eu/publications/29
DOI: 10.1101/2023.09.26.559599
Teams: yPublish - Bioinfo tools
Publication type: Journal
Citation: biorxiv;2023.09.26.559599v1,[Preprint]
Date Published: 27th Sep 2023
Registered Mode: by DOI

Views: 1560
Created: 23rd Oct 2023 at 15:30
Last updated: 23rd Oct 2023 at 15:32


None